SER Blog Innovation & Technology
When academia & industry collaborate to advance AI research
Artificial intelligence promises an abundance of benefits for companies. From optimizing business processes and supporting employees, to identifying business risks early and much more besides, companies see a bright future with AI. Yet implementing AI concepts can be fraught with challenges. That’s where Prof. Dirk Krechel, Prof. Adrian Ulges and their team of researchers at RheinMain University of Applied Sciences come in. The Deep Content Analytics (DeepCA) project develops AI solutions that cater to the needs of companies and employees from the get-go. In our interview, Prof. Krechel gives insights into the project and explains why the integrated approach benefits both research and industry.
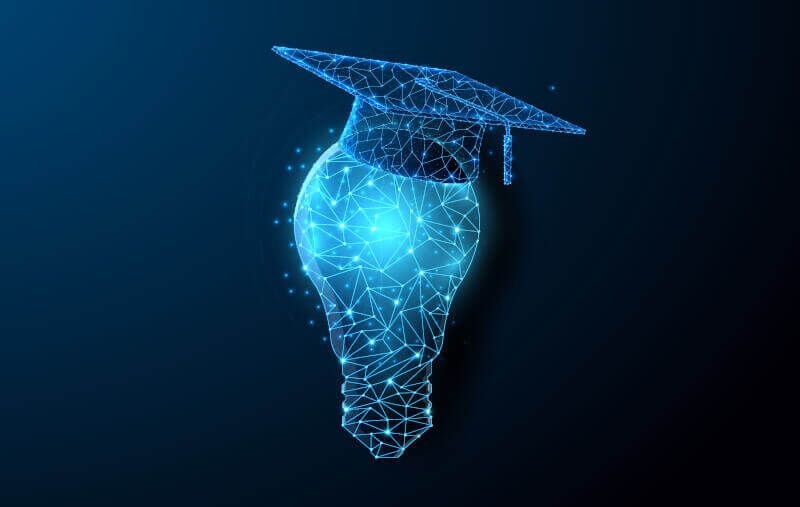
Prof. Krechel, you and your team are probing the field of content analytics. How did this project come about and what are your goals?
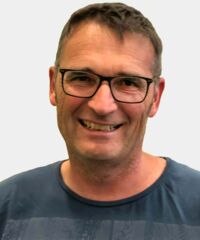
Prof. Krechel: At RheinMain University of Applied Sciences, students of design, information and media get the unique opportunity to work on research and technical questions in direct collaboration with companies in the industry. At present, our focus is on developing typical use cases for combining deep learning technologies with ECM systems. The SER Group is a long-standing patron of the university and our collaboration in the LAVIS (Learning and Visual Systems) working group goes back to 2007. At present, the focus of our partnership is the Deep Content Analytics (DeepCA) research project, funded by the Federal Ministry of Education and Research.
Both sides profit from the relationship: Our students learn about the real-world mandates and typical development processes found at a software vendor, and gain professional experience during their studies. In return, a company such as the SER Group benefits from an influx of academic research to support the development of next-generation software systems.
Can you tell us more about "DeepCA"?
Prof. Krechel: The project name DeepCA is an amalgamation of deep learning and content analytics. Content analytics is all about extracting knowledge from a range of data sources. In most corporate contexts, these sources take the form of systems, databases and applications. Our project mainly looks at unstructured data content found within natural language texts, e.g. documents. Unlike with structured data such as material master data in an ERP system, natural language texts are not so easy to search and analyze using conventional methods. This is where deep learning techniques like natural language processing (NLP) come in. NLP is a hot topic of research right now. The latest findings allow us to analyze natural language texts and improve things like document searches, tagging and categorization.
A major focus for us is on analyzing semantic similarities between passages of text in business documents. The idea is to identify fuzzy similarities that standard keyword searches don’t pick up on. Online search engines are a perfect example of how this kind of similarity search is already being used with great success. Our DeepCA project probes how these technologies can translate into a company context.
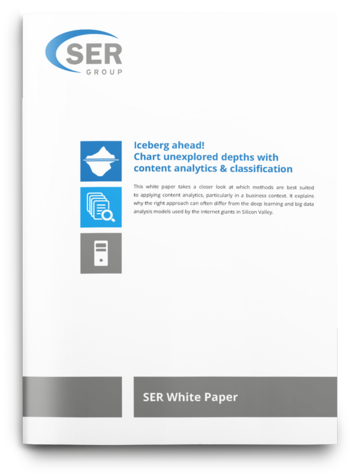
Content analytics in a corporate context
In this paper, you’ll find out how content analytics helps companies gain valuable insights from unstructured information.
Read nowWhy is it important for companies to utilize technologies like deep learning and content analytics?
Prof. Krechel: Take any department in any company and you’ll find almost all accumulate vast quantities of information from various sources daily. Mostly this information is filed in an unstructured way. It’s virtually impossible to find centrally, let alone be used for analysis or in the right business context. It’s annoying for colleagues who have to waste time looking for information, especially if the customer is waiting for an answer. And there’s the additional risk exposure if important information goes missing, like contracts. There’s huge potential to be harnessed, but companies simply aren’t able. They have a valuable pool of data essentially lying dormant. We explore processes that allow this information to be easily tapped in an ECM environment and actively used in workflows.
Can you give a couple of examples from the corporate context to show how these processes work in practice?
Prof. Krechel: The processes help companies in a wide range of areas. You have the classic extraction scenarios, for instance where metadata is mined from unstructured information. Or there’s the semantic search, which enables companies to explore and tap into their internal document pools. The system can significantly improve the results of searches for similar documents relating to a specific business process.
Legal document searches are a prime example. Company lawyers often have to trawl through court judgments to find arguments to back a specific legal position and then apply these to the current situation. Search engines like juris.de use a familiar keyword-matching model. But it’s then up to the lawyer to further analyze the target documents by hand. Paraphrasing, sentence structure and other factors all affect whether a passage from the text supports the argument. A similarity search allows texts to be found without the extra manual effort. Part of our research involves investigating how the proximity of certain words to one another can be used to recognize similar documents. In a business context, this system allows users to do things like search through existing contracts for specific clauses and retrieve all instances where the clauses have been superseded, making them invalid.
Machine maintenance in the industrial sector is another example. An information extraction service developed by us can identify the machine parts described in technical service tickets and recognize the error symptoms. Technical experts can then compare the outcome against related problems and solutions and more swiftly deliver knowledge-intensive technical support.
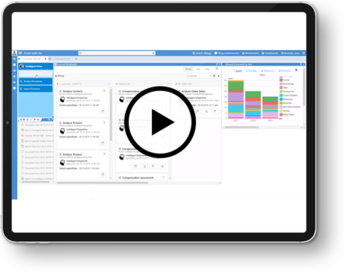
Intelligently share knowledge with Doxis
In our video, you’ll see how to intelligently manage and share knowledge across your company with Doxis to enhance collaboration and shorten your time-to-market.
Watch nowWhy don’t more companies use these kinds of approaches?
Prof. Krechel: AI models need to be trained with suitable data before being deployed for business. A classic example that most people think of here is automated invoice reading. The system learns with every new invoice it reads to continually improve the quality of recognition. But its success is dependent on a sufficiently large volume of learning data — no problem when you have thousands of inbound invoices. But for other types of documents that only exist in smaller quantities, the data cohort is often not large enough. Where this is the case, we have found that few-shot learning scenarios with limited sample data can still produce really good results: Novel artificial neural models begin by learning from conventional search machines, like the Okapi BM25 ranking models used in Elasticsearch. The learned models can then be refined with feedback, for instance provided by employees when searching for company information. It allows companies to exploit the intelligence of large search engines and adapt it to their individual needs. This opens up boundless possibilities for the deployment of AI.
For example, team members can define a business process via a small volume of documents and have the ECM system display similar processes from which they can take over responsibilities, release stages and much more. Not only does this save substantial time that employees would otherwise spend researching and organizing workflows, but it also offers assurance for decision-makers.
What do you personally consider the most exciting thing about deep learning and content analytics?
Prof. Krechel: Actually, all areas of current AI research are incredibly exciting because there’s so much more we have yet to discover. A big motivator for me is how our project ties in with specific use cases for companies. It brings our research findings to life, if you will. Rather than gathering dust on a bookshelf somewhere, they are applied in the real world with genuine benefits for users. This is also how we motivate our students. They get to learn in a practical environment, which fires them up about things like complex algorithms that would be particularly dry in theory only.
So students, universities and companies alike benefit from this kind of collaboration?
Krechel: Exactly. Our funded projects involve regular coordination meetings with partner companies such as the SER Group. Their feedback is an important tool that helps us to evaluate our research findings. And the project leads aren’t the only ones who work directly with the companies: our PhD students are also in close contact with our partners. It’s an opportunity for them to gain professional experience for their future careers. The companies themselves see the value of these partnerships in helping them incorporate the latest research findings into their product evolution. Not only that, but they get to meet highly qualified potential employees with an in-depth understanding of product development. We have a very productive collaboration with the SER Group going back many years. We are already working on follow-up applications for the project and plan to begin investigating concrete use cases for specific sectors, such as banking and insurance. I’m excited to see the fruits of our cooperation in the future!
Find out more about the project:
Prof. Dr. Dirk Krechel
RheinMain University of Applied Sciences
Fachbereich DCSM, Studiengang Medieninformatik
Haus D Unter den Eichen 5, 65195 Wiesbaden, Germany
dirk.krechel@hs-rm.de | deepca.cs.hs-rm.de
The latest digitization trends, laws and guidelines, and helpful tips straight to your inbox: Subscribe to our newsletter.
How can we help you?
+49 (0) 30 498582-0Your message has reached us!
We appreciate your interest and will get back to you shortly.